23 April, 2025
Very simple to understand: RoPE, 2D-RoPE, M-RoPE
Keep it simple, stupid! Explaining RoPE, 2D-RoPE, M-RoPE in a simple way.
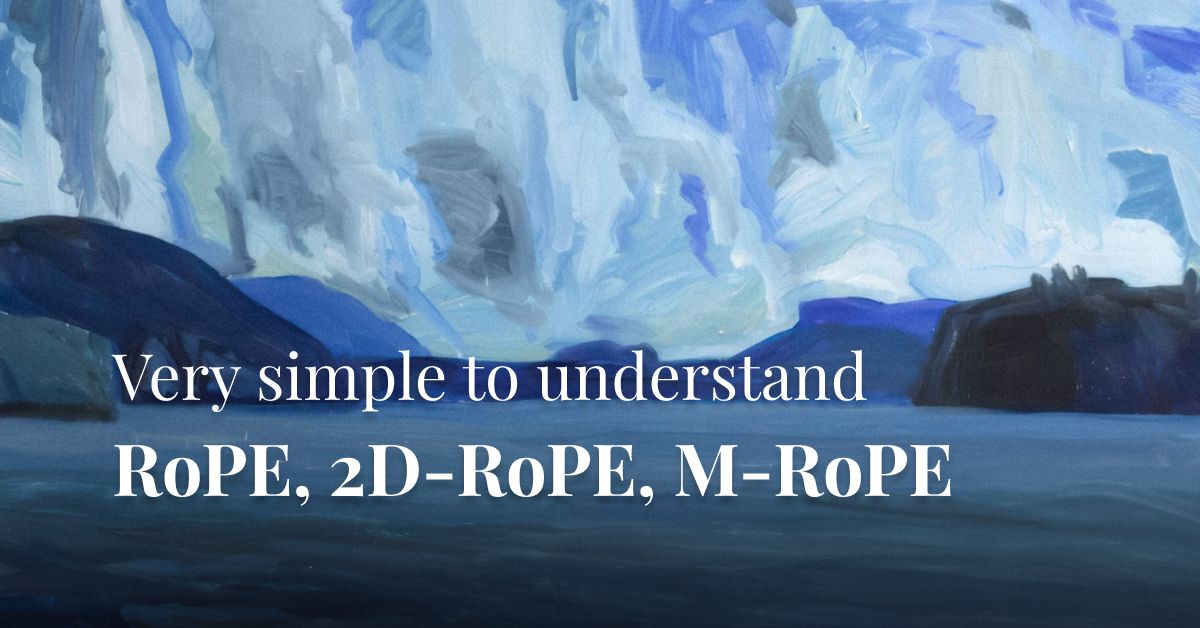
- Why positional embeddings?
- RoPE
- 2D-RoPE
- 2D-RoPE with interleaved frequency
- M-RoPE
- Conclusion
This article offers a simplified explanation of (1D-)RoPE, 2D-RoPE, and M-RoPE, aiming to capture the core ideas concisely.
Motivation? I was struggling to understand these concepts, mostly because my brain functions solely on visualizing things, not weird math equations π
With that in mind, I've structured this explanation to be intuitive and visually oriented. Hopefully, this approach will help clarify these concepts for you.
Why positional embeddings?
Imagine you have 2 language models, one can only process one word at a time, while the other can process all words in parallel.
Now, we have a sequence of words, like "Dog eats food."
- With the first model, the order of input matters, because it must firstly process "Dog", then "eats", and finally "food". But obviously, this is slow and inefficient!
- With the second model, the order of input doesn't matter, so you can throws all the words at once, even out-of-order, like "food", "Dog", "eats". Because the model can process all of them in parallel, it is much faster.
The problem with the second model is that it doesn't know the order of the words. So, we need to add some positional information to the input embeddings.
Now, imagine that instead of words, we N embedding vectors, each of size n_dim
. Let's take an example with 4 embedding vectors, each of size n_dim = 8
and initialized to 1:
1 | 1 | 1 | 1 | 1 | 1 | 1 | 1 |
1 | 1 | 1 | 1 | 1 | 1 | 1 | 1 |
1 | 1 | 1 | 1 | 1 | 1 | 1 | 1 |
1 | 1 | 1 | 1 | 1 | 1 | 1 | 1 |
Now imagine that we apply a positional embedding 0, 1, 2, 3
to each of the embedding vectors. We are also using an imaginary method which simple adds the positional index to each of the embedding vectors. So, the first embedding vector will be 1 + 0
, the second 1 + 1
, and so on.
1 | 1 | 1 | 1 | 1 | 1 | 1 | 1 |
2 | 2 | 2 | 2 | 2 | 2 | 2 | 2 |
3 | 3 | 3 | 3 | 3 | 3 | 3 | 3 |
4 | 4 | 4 | 4 | 4 | 4 | 4 | 4 |
Of course, this is just a simple example to illustrate the concept. In reality, this won't work. So, what do we do?
RoPE
RoPE stands for Rotary Position Embedding. It is a method to encode positional information in the input embeddings of a transformer model.
RoPE works by simply rotating the input embedding vectors in a 2D space. I won't go into the math details, but here is a simple illustration:
The input vector is [x=0, y=1]
, each position rotates the embedding vector by f = 20Β°
, counter-clockwise.
pos = 0 β | pos = 1 β |
pos = 2 β | pos = 3 β |
In real world, we don't just have 2 dimensions, we have n_dim
dimensions. For example, n_dim = 4
:
0 | 1 | 0 | 1 |
0 | 1 | 0 | 1 |
0 | 1 | 0 | 1 |
0 | 1 | 0 | 1 |
So how can we rotate a vector in n_dim
space?
The answer is simple: We split the vector into 2D pairs:
0 | 1 | 0 | 1 | |
0 | 1 | 0 | 1 | |
0 | 1 | 0 | 1 | |
0 | 1 | 0 | 1 |
I will replace each pair with an arrow to simplify the illustration:
β | β |
β | β |
β | β |
β | β |
Now, here is the fun part: We do not rotate each vector by the same angle, because we will quickly end up using up all the angles possible.
The best way to think about it is like a clock π , where for each full rotation of the second hand, the minute hand only rotate a small amount.
In RoPE, the rotated amount is called frequency (noted as f
) and is defined as:
Where i
is the index of the 2D pair (ranging from 0
to d/2 - 1
), and base
is a predefined constant, typically 10000.
To determine the rotated amount for each pair (noted as theta
, ΞΈ
), simply multiply the position index pos
by the frequency f
:
For simplicity, let's assume we still have f = 20Β°
for the first pair, and f = 10Β°
for the second pair:
pos | f = 20Β° | f = 10Β° |
0 | β | β |
1 | β | β |
2 | β | β |
3 | β | β |
As you can see, the first pair rotates faster than the second pair, just like the second hand of a clock rotates faster than the minute hand.
And the same way 3 clock hands are used to represent all the 86400
seconds in a day, we can use the same idea to represent all the n_dim
dimensions in RoPE.
2D-RoPE
So far, we have only discussed RoPE, which is a 1D positional embedding method. It is useful for 1D sequences, such as text.
But what if we want to use RoPE for 2D sequences, such as images?
2D-RoPE is a simple extension of RoPE, where we have 2D position [y,x]
for each input vector:
[0,0] | [0,1] | [0,2] | [0,3] |
[1,0] | [1,1] | [1,2] | [1,3] |
[2,0] | [2,1] | [2,2] | [2,3] |
[3,0] | [3,1] | [3,2] | [3,3] |
Going back to the clock analogy, a simple idea is to use 2 clocks, one for the y
axis and one for the x
axis.
To illustrate this, let's double the n_dim
of the initial example, so we have n_dim = 8
:
0 | 1 | 0 | 1 | 0 | 1 | 0 | 1 |
0 | 1 | 0 | 1 | 0 | 1 | 0 | 1 |
0 | 1 | 0 | 1 | 0 | 1 | 0 | 1 |
0 | 1 | 0 | 1 | 0 | 1 | 0 | 1 |
Which is now represented as 4 pairs of 2D vectors:
β | β | β | β |
β | β | β | β |
β | β | β | β |
β | β | β | β |
The idea is to futher split the vector into 2 sections, one for the y
axis and one for the x
axis:
for Y pos | for X pos | |||
β | β | β | β | |
β | β | β | β | |
β | β | β | β | |
β | β | β | β |
Let's say the list of positions for these 4 vectors are:
[0, 0]
[0, 1]
[1, 2]
[1, 3]
Using a set of f = 40Β°
and f = 20Β°
, we can rotate the vectors for each section independently like this:
Y | f = 40Β° | f = 20Β° | X | f = 40Β° | f = 20Β° | |
0 | β | β | 0 | β | β | |
0 | β | β | 1 | β | β | |
1 | β | β | 2 | β | β | |
1 | β | β | 3 | β | β |
As far as I know, this 2D-RoPE method is used in the vision encoder of Llama 4 model.
2D-RoPE with interleaved frequency
In the previous example, we used the same f = 40Β°
and f = 20Β°
for both axes. But what if we want to use different frequencies for each axis?
For example, with Mistral's Pixtral model:
- We firstly create a list of frequencies for all pairs of 2D vectors, example:
40Β°, 30Β°, 20Β°, 10Β°
- Then we interleave the frequencies for each axis, so we have
40Β°, 20Β°
for they
axis and30Β°, 10Β°
for thex
axis.
Y | f = 40Β° | f = 20Β° | X | f = 30Β° | f = 10Β° | |
0 | β | β | 0 | β | β | |
0 | β | β | 1 | β | β | |
1 | β | β | 2 | β | β | |
1 | β | β | 3 | β | β |
The cool thing is that instead of constructing a list of frequencies (for example, 40Β°, 30Β°, 20Β°, 10Β°
) then select the odd/even f
, you can tweak the n_dim
value and the scale of f
to get the same result. See this PR to understand how I did it.
M-RoPE
M-RoPE stands for Multimodal-RoPE. It is firstly introduced by Qwen2VL.
M-RoPE extends the idea of 2D-RoPE, but now we have more than just 2 dimensions per position. For example, we can have 3D [time, y, x]
or even more dimensions.
The key idea is instead of splitting the embedding vector into 2 sections, we split it into... yes, you guessed it, n
sections, where n
is the number of dimensions per position.
If you look closely at the config.json of Qwen2VL, you will see a config called mrope_section
, which contains 3 number. Each number represents the number of 2D pairs for each section.
"rope_scaling": {
"type": "mrope",
"mrope_section": [
16,
24,
24
]
},
To make it easier to understand, let's take a simple example with embedding of n_dim = 8
, we end up with 4 pairs of 2D vectors:
β | β | β | β |
β | β | β | β |
β | β | β | β |
β | β | β | β |
Assuming that our mrope_section
is [1, 1, 2]
, we can split the embedding vector into 3 sections:
β | β | β | β | ||
β | β | β | β | ||
β | β | β | β | ||
β | β | β | β |
Then, RoPE is applied to each section independently, using the same method as explained in 2D-RoPE.
β | β | β | β | ||
β | β | β | β | ||
β | β | β | β | ||
β | β | β | β |
Conclusion
In this post, we explored positional embedding techniques using a visual-first approach.
Hopefully, visualizing these methods as rotations and dimension splits makes the underlying concepts of RoPE, 2D-RoPE, and M-RoPE more intuitive and easier to grasp, especially compared to diving straight into complex mathematical formulas.